Master thesis topics
To have more information on any of the proposals, please contact either Prof. Ville Kyrki or one of the advisors indicated in the proposal of interest.
At present, the following master thesis proposals are available in the group:
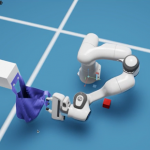
Master Thesis on “Imitation Learning from Human Demonstrations for Manipulation of Deformable Objects”
The thesis focuses on learning-based dynamic modeling of deformable objects for manipulation tasks.
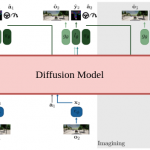
Master Thesis on “Predictive World Models for End-to-End Autonomous Driving”
This research aims to explore whether learning and leveraging world models can also be beneficial in visual representation learning for autonomous driving in a closed-loop settings.
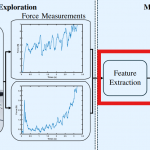
Master’s Thesis Topic on Discriminative Filtering/Feature Extraction for Classification of Tactile Signals
We are currently seeking for a motivated and talented master’s student to work on discriminative filtering and feature extraction for classification of tactile signals.
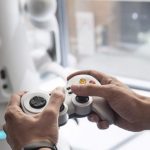
Master Thesis on “Teleoperation and Assistive Systems”
We are currently seeking a motivated and talented master’s student to work on developing a Teleoperation and Assistive system for robotic systems as part of their master’s thesis.
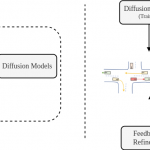
Master Thesis on “Data-Driven Diffusion Models for Enhancing Safety in Autonomous Vehicle Traffic Simulations”
This thesis aims to develop a data-driven diffusion model that elevates realism and controllability in simulations and intricately models the complex interactions between multiple agents for safe planning
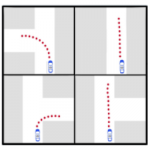
Master Thesis on “Deep Imitative Models for Safe Planning of Autonomous Driving”
The goal of this thesis is to devise an algorithm that combines the advantage of both IL and MBRL for robust and safe planning for autonomous driving. In this context, the thesis is expected to include implementations of IL and MBRL algorithms and fuse them for planning tasks.