Exploiting Object Physical Properties for Grasping
In robotic manipulation, robots are required to interact with, and adapt to, unknown environments and objects. In order to successfully accomplish these tasks, robots need to identify various properties of the objects to be handled. For these reasons, identifying object models that can represent the properties of objects has become a crucial issue in robotics. Many object-modeling approaches have focused on object shape and geometry by utilizing vision. However, other
physical properties also play an important role in characterizing object
behavior during interaction and handling. In particular, mass, elasticity, surface properties such as surface friction, texture, and roughness are vital for manipulation planning.
Various methods have been proposed to learn object surface properties from vision or haptic feedback or the combination of both vision and haptic cues similar to human perception. However, most published works assume the surface properties to be identical across the whole surface of the object. This assumption does not hold for many real objects, since objects often consist of multiple materials.
To address this, we propose a method to estimate/ simulate the properties of a multi-material object by combining a visual prior with haptic feedback obtained while performing haptic exploration on the object in the real world/ simulation. This estimated/ simulated information can be then exploited for manipulation tasks such as grasp planning.
For the first step, we focus on one property, the surface friction coefficient, but the proposed method could be applied to other properties such as texture and roughness. The approach is based on the assumption that visual similarity implies similarity of surface properties. By measuring a property directly using haptic exploration over a small part of the object, the joint distribution of visual and haptic features can be constructed. Using the joint distribution, the measurement can then be generalized over all parts of the object that are visible. The inference allows recovering both the expected value of friction for each part as well as a respective measure of prediction confidence.
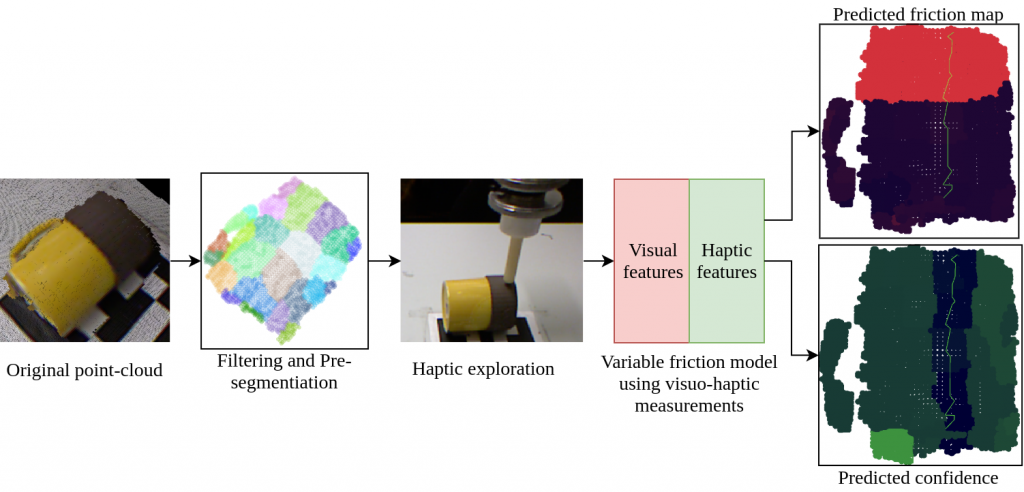
People involved
- Tran Nguyen Le (tran.nguyenle@aalto.fi), Doctoral Candidate
- Fares J. Abu-Dakka (fares.abu-dakka@aalto.fi), Research Fellow
- Ville Kyrki (ville.kyrki@aalto.fi), Professor
Project updates
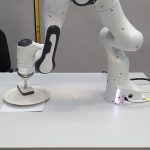
SPONGE: Sequence Planning with Deformable-ON-Rigid ContactPrediction from Geometric Features
Abstract: Planning robotic manipulation tasks, especially those that involve interaction between deformable and rigid objects, is challenging due to the complexity in predicting such interactions. We introduce SPONGE, a sequence planning pipeline powered by a deep learning-based contact prediction model for contacts between deformable and rigid bodies under interactions. The contact prediction model is trained […]
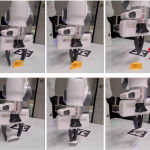
Deformation-Aware Data-Driven Grasp Synthesis
Abstract: Grasp synthesis for 3-D deformable objects remains a little-explored topic, most works aiming to minimize deformations. However, deformations are not necessarily harmful—humans are, for example, able to exploit deformations to generate new potential grasps. How to achieve that on a robot is though an open question. This letter proposes an approach that uses object […]
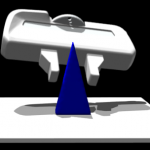
Towards synthesizing grasps for 3D deformable objects with physics-based simulation
Grasping deformable objects is not well researched due to the complexity in modelling and simulating the dynamic behavior of such objects. However, with the rapid development of physics-based simulators that support soft bodies, the research gap between rigid and deformable objects is getting smaller. To leverage the capability of such simulators and to challenge the […]
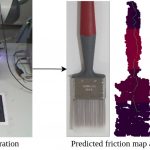
Probabilistic Surface Friction Estimation Based on Visual and Haptic Measurements
Accurately modeling local surface properties of objects is crucial to many robotic applications, from grasping to material recognition. Surface properties like friction are however difficult to estimate, as visual observation of the object does not convey enough information over these properties. In contrast, haptic exploration is time consuming as it only provides information relevant to […]