Robot Control
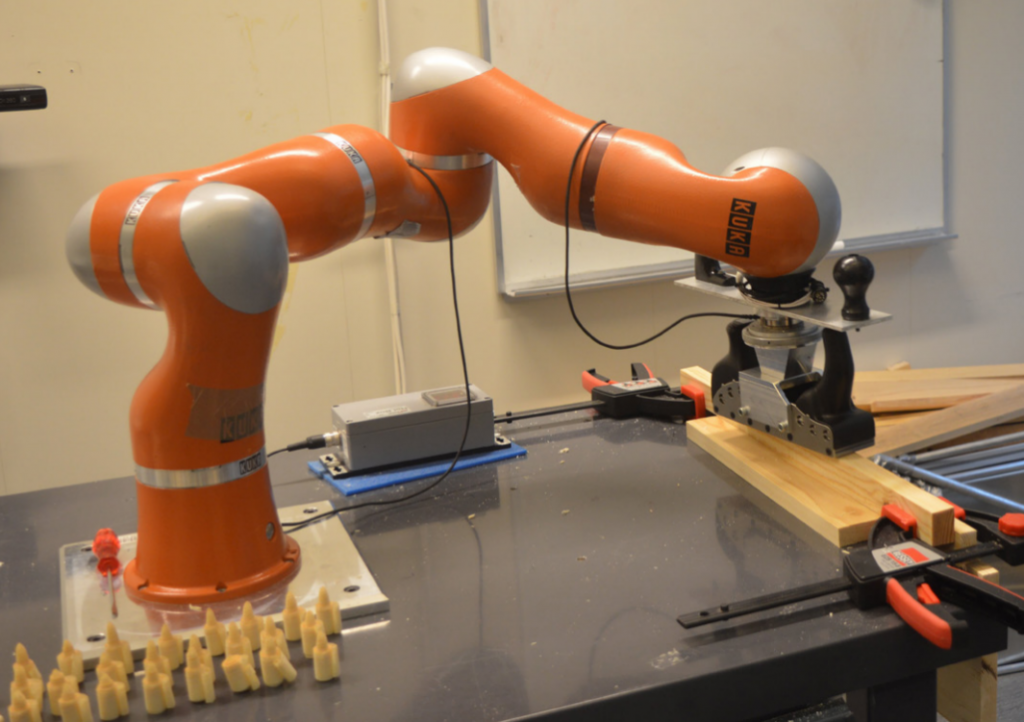
Robotic tasks in real-world applications generally involve uncertain, stochastic and dynamic environments. Pre-programming based solutions either do not work or give unsatisfactory results in such environments. This requires to generate cautious control strategies that provide optimum actions to perform the desired task while considering the effects of the uncertainties in the environment.
Robot control aims to develop learning and optimization based control strategies which consider not only the uncertainties in the environment but also other constraints such as safety, physical limits of the actuators and user-defined preferences.
What we do
- Developing constrained model predictive control (MPC) based solutions with probabilistic state constraints for safe operation;
- Developing safe and efficient exploration policies extending the ergodic control for the exploration in reinforcement learning.
Current Projects
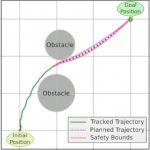
Safe Model Predictive Control
Safe Model Predictive Control (Safe MPC) aims to ensure that a physical system’s safety constraints are satisfied with high probability. Our research is on extending constrained MPC methods to cope with probabilistic safety constraints. We further research modeling uncertainty of dynamics to ensure safe exploration when combined with safety constraints learned in simulation, and learning powerful data-efficient surrogate models for complex dynamics.