Safe Model Predictive Control
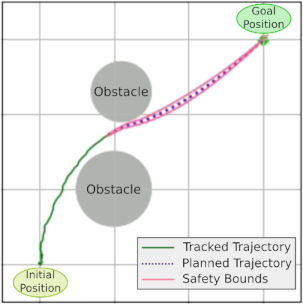
In many real-world applications, robots are involved in uncertain, stochastic and dynamic environments, and required to satisfy some constraints such as collision avoidance, physical limits of the actuators, some user-defined preferences, etc. Within this context, “safety” can be defined as the stability of the control policy that satisfies the constraints considering also the uncertainties on them.
Thanks to model-based techniques, we can be explicitly aware of the safety requirements and optimize our control policy in such a way that the performance is not overly restricted while meeting the safety requirements.
Safe Model Predictive Control (Safe MPC) aims to ensure that a physical system’s safety constraints are satisfied with high probability. Our research is on extending constrained MPC methods to cope with probabilistic safety constraints. We further research modeling uncertainty of dynamics to ensure safe exploration when combined with safety constraints learned in simulation, and learning powerful data-efficient surrogate models for complex dynamics.
People involved
- Gökhan Alcan (gokhan.alcan@aalto.fi), postdoctoral researcher
- Ville Kyrki (ville.kyrki@aalto.fi), professor