Sim-to-real transfer in reinforcement learning
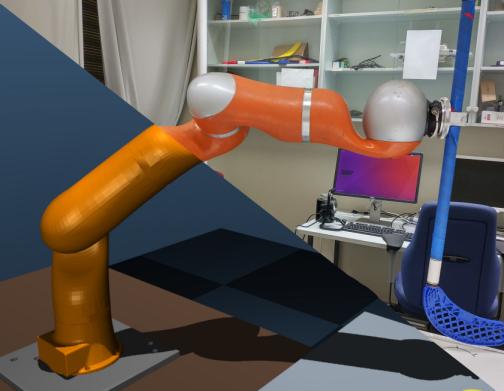
Getting robots to autonomously learn to perform various tasks is often a long-term process, during which the robot’s exploratory actions can be unpredictable and potentially dangerous to the surrounding environment and to the robot itself. To mitigate the risk of hardware damage and to speed up the learning process, initial phases of learning are often performed in simulation. Ideally, we would like to deploy the learned policy directly on the real robot (a zero-shot transfer scenario — however, due to simulation inaccuracies, this approach often turns out to be difficult or nearly impossible. In such cases, either the characteristics of the new environment have to be learned (system identification) or the trained policy has to be adjusted to perform well in the new environment (domain adaptation).
Our research in sim-to-real transfer includes both zero-shot transfer through domain randomization, as well as domain adaptation approaches
People involved
- Karol Arndt (e-mail), doctoral candidate