Evolving-Graph Gaussian Processes poster at the Time Series Workshop at ICML 2021
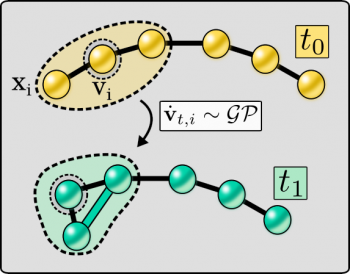
We are happy to announce that our work “Evolving-Graph Gaussian Processes” has been presented at the Time Series Workshop at ICML 2021.
This work extends the current SotA of Graph Gaussian Processes (GGPs) to dynamic graphs and asses the performance of the proposed evolving-Graph Gaussian Process (e-GGP) in two simulated tasks where deformable objects are represented as a graph that evolves over time.
The Open Source code can be found at
Dark Mode
evolving-ggp (this link opens in a new window) by aalto-intelligent-robotics (this link opens in a new window)
Evolving-Graph Gaussian Processes.